B2B Commerce: How to Build a Data-Driven Content Strategy that Proves ROI
Jul 02, 2021
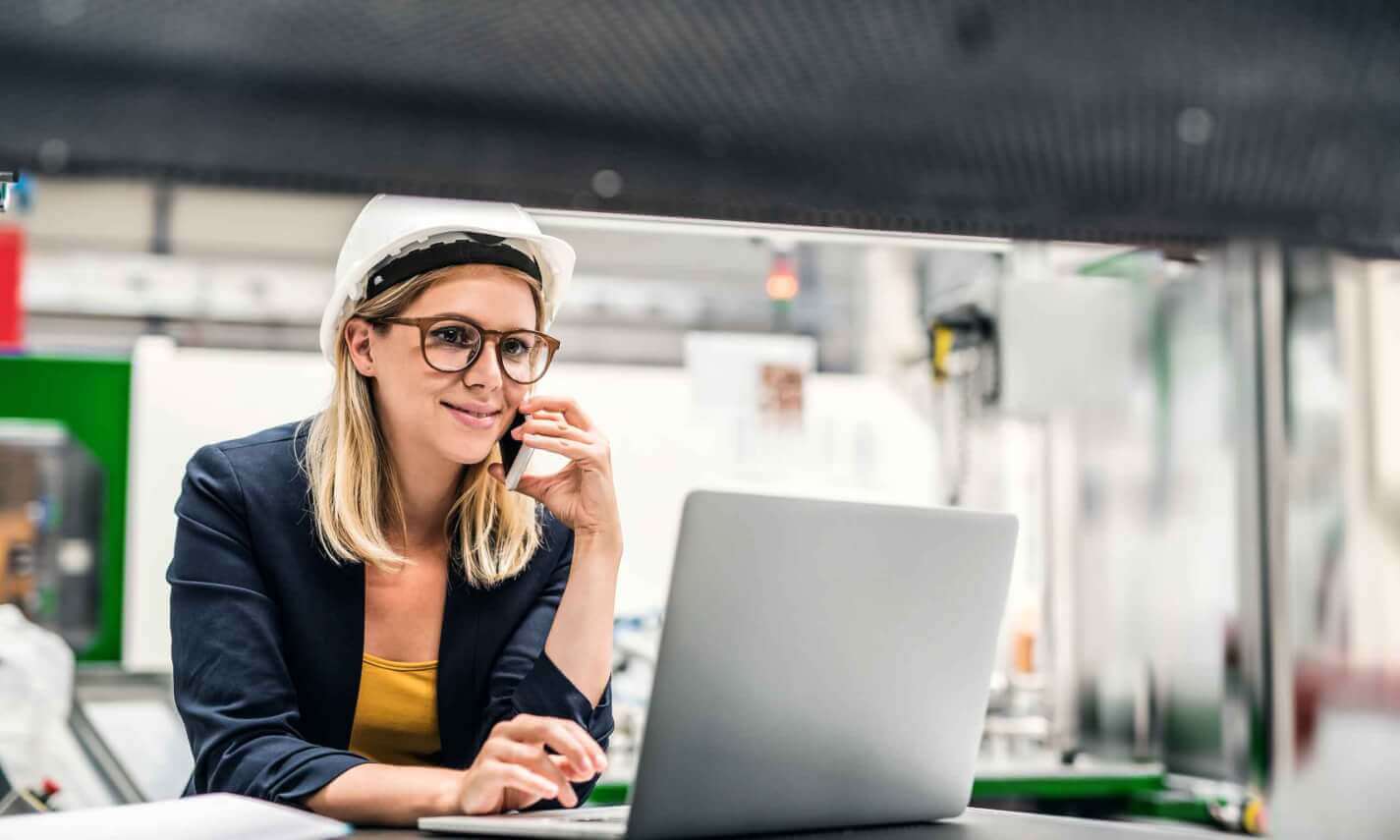
The 4th industrial revolution is well on its way. Technologies like AI are changing the landscape of industries such as manufacturing and supply chain management in more ways than one.
Already now, we see better analytics, more frequent use of robots, and more sophisticated use of ever-growing databases. But more is to come.
It is a known fact that automation in manufacturing has increased productivity and replaced many tedious jobs with the help of industrial robots. But since 2017, a new kind of robot is entering the workforce.
Collaborative robots, or cobots for short, now work alongside humans, assisting them in or taking over tasks that are either hazardous, strenuous, or tedious. Not only do cobots create safer, more efficient work environments, but they also do so without eliminating jobs.
The main benefits of cobots are:
The global cobot market is expected to have a compound annual growth of 58.79%, reaching $9.13 billion by 2024.
As more and more sensors are embedded in every part of a supply chain, and AI and machine learning are applied to the growing amounts of data, we see an emergence of a new, smarter supply chain.
Together with Big Data, machine learning algorithms have made it easier to identify real-time purchasing behaviors. In turn, manufacturers and shipping companies can make more accurate demand forecasts, adjust the prices of their products, and improve overall operations.
Predictive maintenance is another area where AI is decreasing costs and improving product quality and safety in the workplace. Real-time data such as equipment usage, technical specifications, the environment of operations, and past maintenance is collected to schedule maintenance of the machine before a failure occurs.
Not only does this extend the lifetime and reliability of the equipment, but it also makes the planning of spare parts purchases more efficient.
Despite the multitude of advantages, only 30% of companies are using predictive analytics today, with about 90% planning to incorporate it in the next ten years.
How to get the right product at the right place and at the right time is an ongoing dilemma in warehouse management. And cognitive inventory management might just be the solution.
Cognitive inventory management can harness data from a lot of different systems, accounting for stock in, stock out and stock remaining, as well as order volatility, demand volatility, and more. The more the system is used, the better it will perform.
In some cases, even data such as items added to carts, but not yet purchased, products saved in wishlists, and also data from a customer’s cursor movements are used to predict what the customer will likely order. Amazon has been at the forefront of experimenting with such data.
As a result, companies can eliminate out-of-stock scenarios, minimize returns, better forecast demand for their product, and overall reduce capital tied in excess stock.
Chatbots in procurement can elevate database queries from simple searches to conversations between the procurement team and the vastly growing procurement databases.
Thanks to Natural Language Processing (NLP), AI chatbots are can answer complex questions and thus shorten the time procurement teams spend on combing through the databases for information. This way, the employees can focus on more value-adding tasks.
Given enough information about prior purchases, preferred suppliers, real-time availability of products, etc., teams can also use AI chatbot to order products more quickly and with less unnecessary research.
Digital twin as a concept was coined over a decade, but it is only with the rise of IoT and AI that companies have been able to take full advantage of this technology. By using sensors, a virtual copy of a physical object is created, which can be further used to run tests and simulations using machine learning algorithms.
According to a report from Gartner, 24% of companies that already have IoT technology in-house, use the digital twin for predictive maintenance and optimization, manufacturing process redesign, prototype tests, or problem-solving. The lessons learned from the virtual tests can later be applied to the original objects with much less risk and higher ROI.
Another benefit of digital twin technology is mobility. Tests of equipment can be done miles away from its location, making it perfect for industries like aerospace, oil and gas, and defense.
The automobile industry has also embraced the digital twin technology. Using a digital twin of a car makes it possible to identify possible problems and technical failures before the production process even begins. This saves time and money in an otherwise expensive development process.
In many ways, AI is still in its infancy. We expect to see exponential growth in this field, which means more opportunities for companies in all industries. For manufacturing and supply chain management, AI might even mean an industry-standard technology.